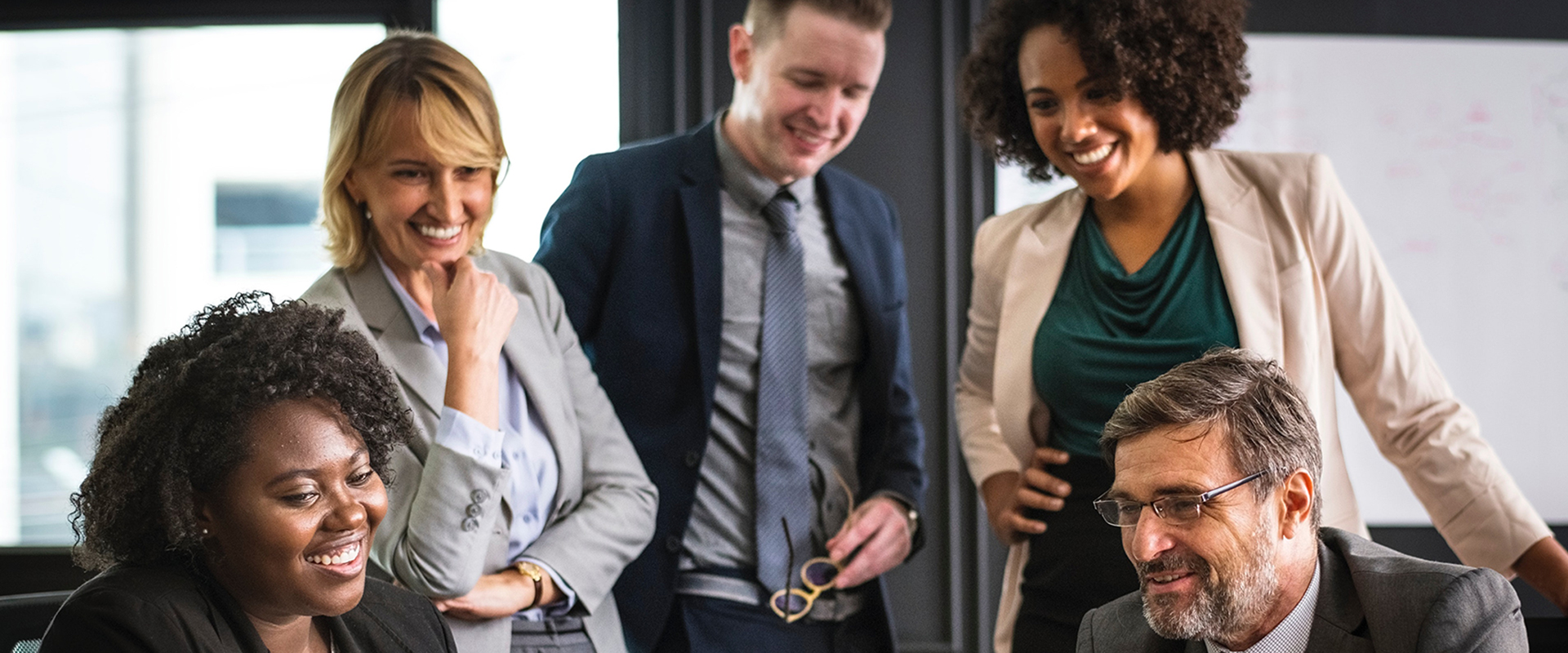
Predicting Churn / Attrition
Churn Prediction is a use case which is useful across verticals such as Retail to Telecommunication. By being able to identify revenue generating customers who could churn ahead of time and being able to retain them in your company by pushing offers and promotions to incentivize them would mean that your Customer Lifetime Value (CLV) increases as well as increasing customer “stickiness” towards your brand.
"Increase Customer Stickiness with the use of Smart Analytics."
If we were to consider a sample use case for Churn Prediction from the Telecommunications domain we could detail our approach to the problem as follows. When considering your customer base, it is most likely that you will have a Revenue Generating Base (RGB) as well as a Non-Revenue Generating Base (NRGB). Typically, you would be interested in retaining your RGB as they are more important from the perspective of Revenue Generation. The segmentation of RGB versus NRGB can be done by analyzing revenue data and the segments can be defined by the business users as the proportion of users they wish to consider as RGB. A further segmentation could be done on whether the users are prepaid users or postpaid users which would help the business team to carry out campaigns to retain the customers once actionable insight regarding who will churn is derived.
In Telco’s, for developing reports for business users on KPIs there is usually an Enterprise Data Warehouse (EDW) where CDR (Call Data Record) data is populated from mediation systems which get Voice Call data, SMS traffic, Data traffic, MMS traffic, Value Added Services (VAS) data etc. from switches. After identifying the relationships between the fact and dimension tables in the EDW, variables which are dynamic and time-varying in nature are derived from the EDW such as off-network voice calls, SMSs, MMSs, as well as voice calls in the network including SMSs, MMSs, VAS used as well as recharges in the case of prepaid customers.
Depending on the businesses definition for the churn period the model can be developed using advanced machine learning models until a reasonable classification accuracy of churn versus not churn is obtained which is agreed upon both by the business and the development team. Afterwards, the model can be operationalized in the production environment and integrated with the businesses Customer Relationship Management (CRM) system to make sure that RGB customers are incentivized to stay on the network if they are flagged as potentially tending to churn based on their usage details.