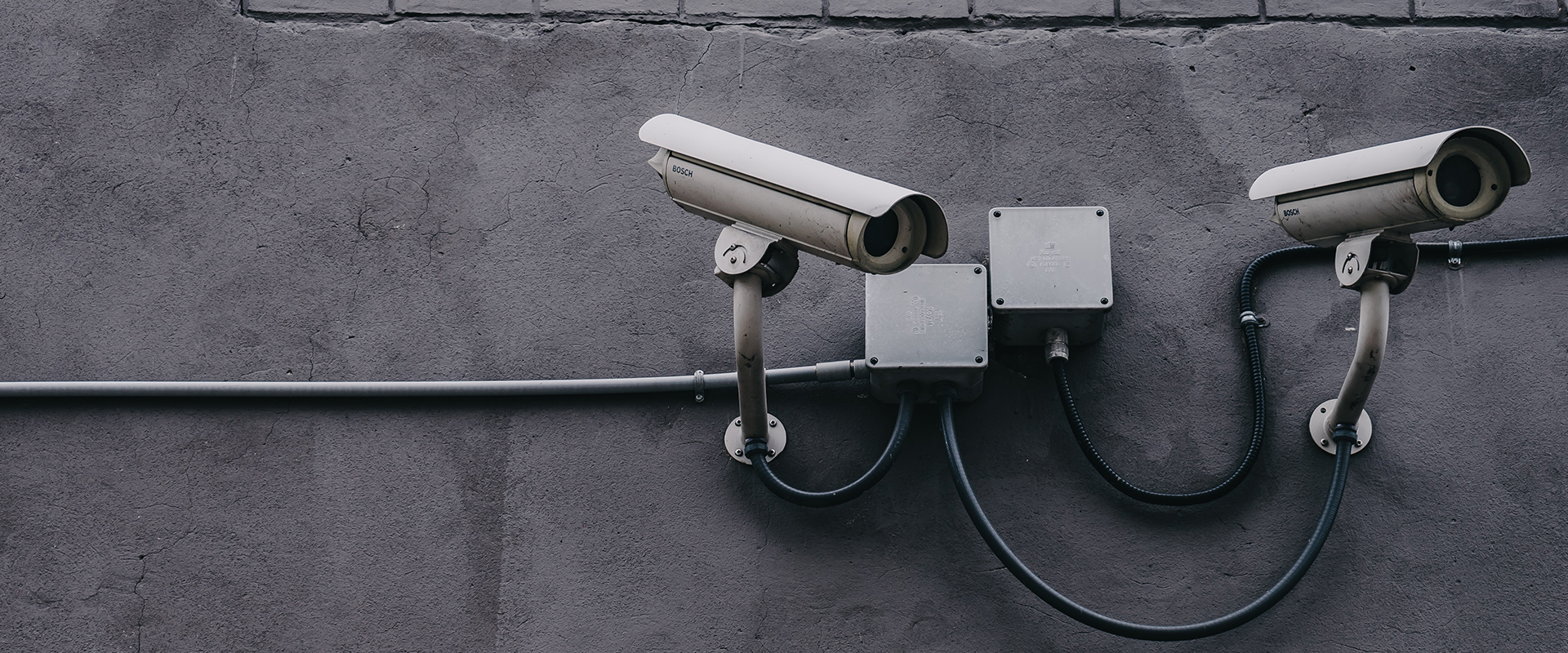
Detecting Fraud / Outliers
The Association of Certified Fraud Examiners estimates that the typical organization loses approximately 5% of its annual revenue each year due to fraud.When this estimate is applied to the expected Gross World Product in 2016 this amounts to a staggering 3.8 million US dollars of global revenue lost due to fraud. With the volume and velocity of transactions being carried out in a typical business continuing to increase it is not humanly possible for the approvers of these transactions to scrutinize each purchase order with the attention it ideally deserves.
Use Intelligent Fraud Detection to mitigate the risk to your company's finances as well as reputation.
In advanced analytics, problems such as fraud detection are usually framed as classification problems or as unsupervised learning problems such as anomaly detection.For example consider about credit card payment fraud detection. This classification problem involves creating models that have enough intelligence in order to properly classify transactions as either legitimate or fraudulent, based on transaction details such as amount, merchant, location, time and others.
Financial fraud still amounts for considerable amounts of money. Relying exclusively on rule-based, conventionally programmed systems for detecting financial fraud would not provide the appropriate time-to-market. This is where Machine Learning shines as a unique solution for this type of problem.
Cogniata can help you build state of the art fraud detection systems utilizing our extensive knowledge across domains such Retail, Telecommunications and BFSI to minimize and mitigate the losses incurred by revenue bleed due to fraud. Our expert team of Data Scientists and Domain Experts will be able to build bespoke machine learning models so that enterprises are able to communicate that they have ensured an additional layer of security to safeguard their business from the different type of frauds which could arise across the complex environments they operate in.