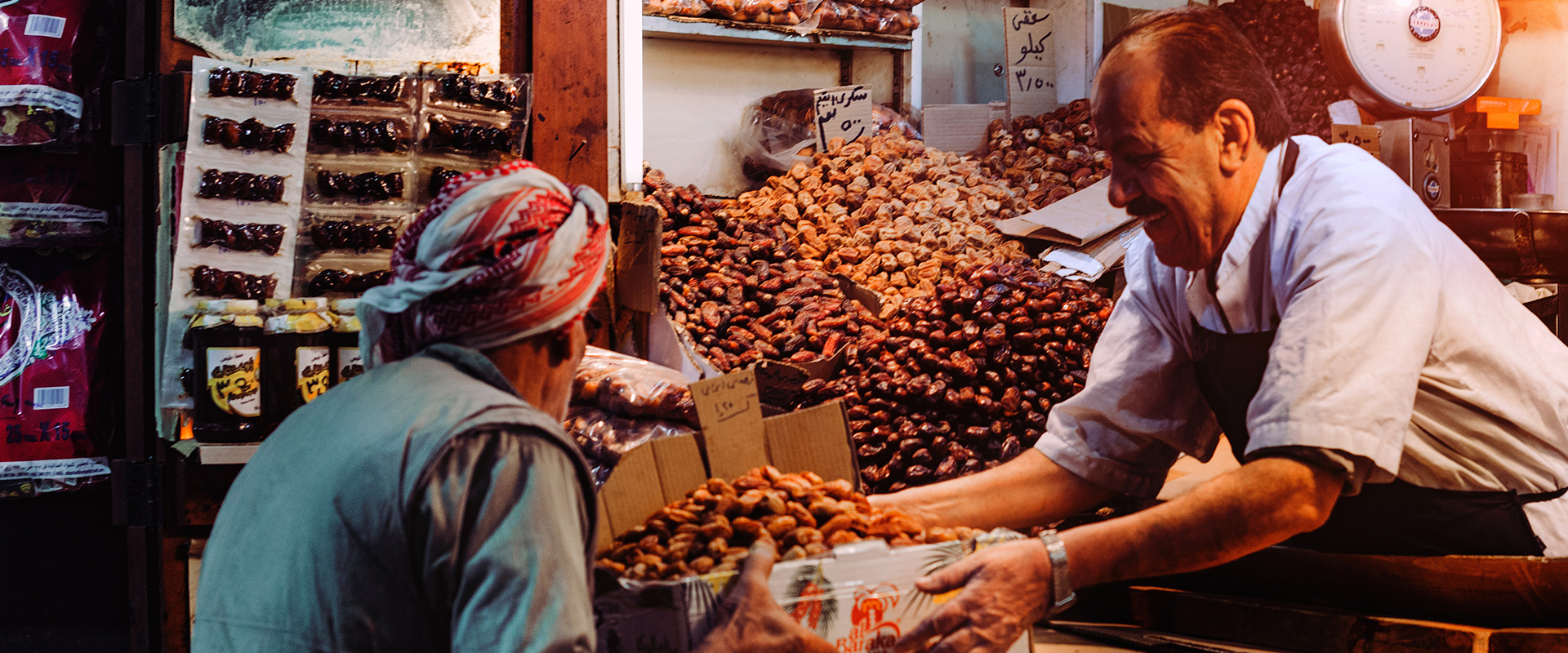
Customer Segmentation
Irrespective of whether a company’s products and services are digitally based or not, direct interaction with customers is increasing, across both digital and physical platforms while customers are increasingly willing to surrender more information to companies they choose, in return for relevant and useful products, services and content.
In order to appeal to customers, it is needed to understand the concept of the market of one where the customer feels that he is being treated as a unique individual and where products, services and content are customized for his individual needs. Customer segmentation helps achieve this by dividing markets into smaller segments of potential customers with similar characteristics who are likely to exhibit similar purchase behavior. This segmentation depends largely on who is most likely to use your product, and the cost of reaching and acquiring those potential customers to use your product.
20% of customers account for 80% of turnover according to Pareto's Law.
Segmentation can be done in various ways: Physical Segmentation, Socioeconomic Segmentation, Behavioral Segmentation and Benefit Segmentation. AI and Machine Learning techniques along with heuristics models such as RFM (Recency, Frequency, Monetary) can be used to achieve this. By efficient segmentation, a business can maximize its promotion spend and increase customer loyalty as well increase conversions.
In order to do customer segmentation we use feature engineering to derive variables with predictive power using statistical techniques such as variable transformation, feature scaling and dimension reduction using Principal Component Analysis (PCA) after which the data is segmented using a clustering technique such as K-Means or Self-Organizing Maps to identify the hidden segments within the data in order to push personalized promotions and content.